So recently I got interested in Chess, and by recently I mean 6 months ago. I learned some important beginner’s lessons along the way and I planned to document them in non-technical language in this article.
I’m assuming you know the very basics, like how the pieces move and what castling is, which is exactly how much chess I knew when I started. If not, go outside (on the internet) and learn the basics. Then create an account on lichess.org or chess.com and play around 10 games with other people. Then come back to this article.
So assuming that we’re on the same page, let’s start!
Stages of the game
A chess game can be visualized to happen in three stages; Conveniently–start, mid and end game. You can be mindful of what stage the game is in as there are certain things you can do in each stage to give yourself a slight edge in the game.
When you start, you try to develop the pieces as quickly as possible and castle. That means all pieces are ready to jump into action; whether attack or defence, and your king’s safety is ensured.
Midgame, depending on your position, you exchange pieces and try to get a better position. Make sure you’re either up material (pieces or pawns), or you have a better hold of the position and control more space on the board.
Endgame is when both sides go all out using whatever positional advantages they’ve created previously. It is also common for endgames to just have king and pawns, maybe a minor piece as well. In this case, both sides try to push their pawns and promote them to Queens (or any other piece but usually the Queen, and the first side to do it usually wins).
Pre game
Know value of the pieces: Not all pieces are as valuable. Pawns are unit value, that is 1. Knights and Bishops are 3, Rooks are 5 and Queen is 9 units each. When exchanging pieces, these values should be taken into account. It generally doesn’t make sense to sacrifice a Rook for a Bishop. Of course, there are times when you’ll want to do it (especially if you have a forced checkmate in place).
The value of your pieces changes depending on your position and the stage of the game. You can read about it on Wikipedia but you will also learn these things intuitively once you have enough games under your belt.
Starting tactics
Castle early, ensure king safety before anything: I used to frequently go for material (i.e. trying to win pawns or pieces) and space before castling which generally doesn’t end well. Once your opponent is done developing their pieces and castling, you’ll end up playing a very defensive game if your king is in the center of the board with undeveloped pieces.
Develop pieces as early as possible: Move all pieces out of their initial position, ready for attack or defence and connect rooks early in the game.
Don’t move the same piece twice in opening: In general, you should avoid moving a piece more than once in the opening as the goal is to develop pieces fast. Of course, exceptions are when your piece is attacked or if the other side offers a gambit (a pawn or minor piece sacrifice to gain positional advantage).
Develop Knights before Bishops: Another general rule of thumb as both Knights can develop and simultaneously control central squares which is usually a good idea.
Rooks work better when connected: Rooks are developed when they’re connected (both Rooks are defending each other). Another common tactic is to double rooks on an open file (a column on a Chess board without pawns) to mount a powerful attack.
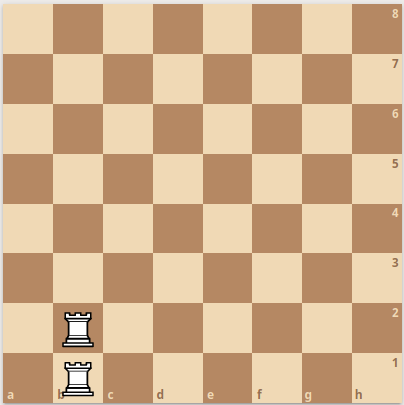
Aim for center control: It is generally a good idea to control the center four squares of the board. There are exceptions, however, as with certain openings we can aim for control over one side of the board and continue our attack from there.
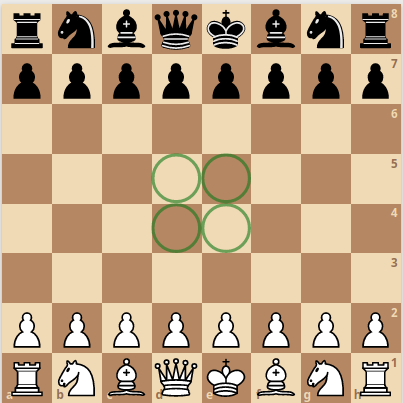
General tactics
Don’t keep pieces in pin: If your piece or pawn is pinned, meaning a more valuable piece is behind it, it is good to unpin the piece (move the piece that’s behind it). This is especially true with king. You don’t want your king to be on the same file as your opponent’s Rook or Queen, and same diagonal as your opponent’s Bishop. If the other side brings their Rook to the same file as your King or Queen, move it away even if there are pieces in between.
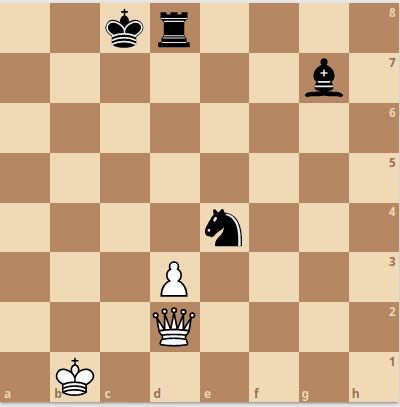
A piece that has moved a lot is worth more than an unmoved piece: If your knight has been moving around the board, it is generally in your opponent’s favour if they manage to exchange your active piece for their dormant piece.
A piece that covers more area is worth more than a piece that covers less area: Similar to a previous one, if your Bishop covers and controls a beautiful diagonal, your opponent might want to get rid of it by exchanging their unused piece for your beautiful Bishop. Try to protect your active pieces.
If you control more space, don’t trade pieces: If your pieces control a lot of space on the board, you should avoid trading pieces.
If you’re crammed, exchange pieces to create room: Conversely, if you’re crammed by your opponent, try forcing exchange of pieces and pawns to create some room for your pieces to develop.
If defending, defend a piece as many times as it is attacked: If you want to defend a piece or pawn, defend it as many times as it is attacked.
If attacking, attack a piece as many times as it is defended plus one: If you’re the one attacking, mount an attack such that the other side runs out of ways to defend.
Two pieces are better than one piece: It generally doesn’t make sense to sacrifice a Bishop and a Knight for a Rook, or two Rooks for a Queen. It is, generally speaking, better to have two pieces instead of one (use the point value of pieces to decide if the exchange makes sense, but remember that active pieces are more valuable than passive ones).
Avoid back rank issues: If you don’t defend the last rank (where your king usually resides), a Rook from your opponent might simply deliver a checkmate (since the pawns in front of your king might be unmoved leaving no room for your king to escape).
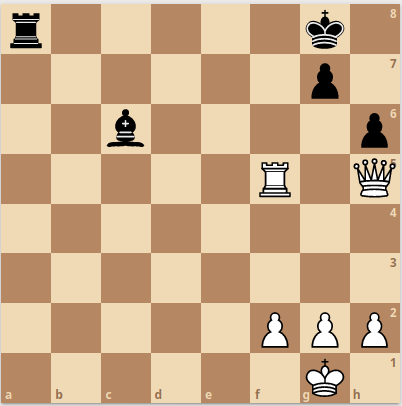
Capture with pawns towards the center: Generally, if you capture with a pawn, you want to capture towards the center of the board if that’s an option.
Knights belong in the center of the board: It is usually not the best idea to move the Knight to a corner of the board as they cover fewer squares from there. They say Knights on the rim are dim
. This applies to most pieces but especially the Knights.
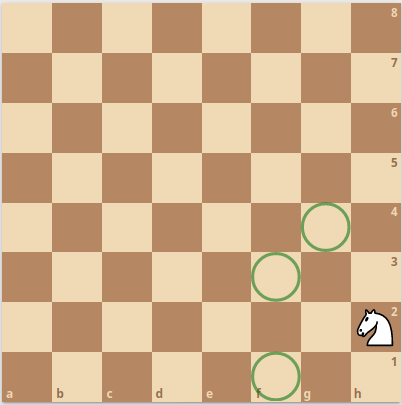
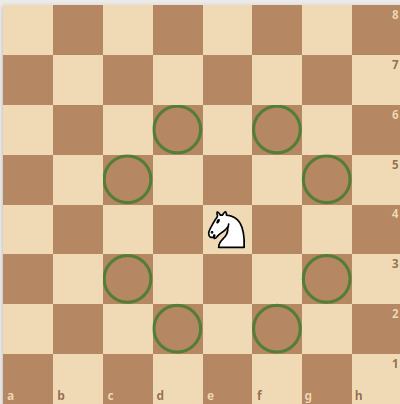
Avoid creating pawn islands: An isolated pawn is one that isn’t connected with your other pawns. Such pawns are liabilities and weaknesses, and hence should be avoided (or sacrificed for positional advantages). In general, try to have as few pawn islands as possible.
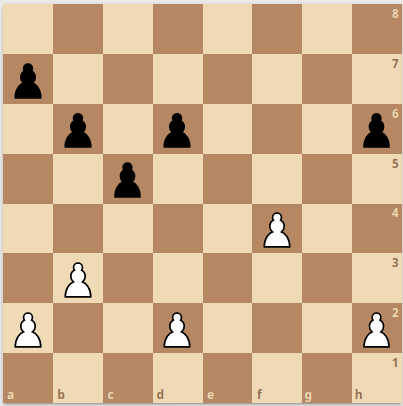
Avoid doubled pawns: Doubled pawns are two pawns on the same file. As with pawn islands, they are weak and can become a liability in the endgame. It is said that a Rook’s favourite meal is a doubled pawn
and is best to avoid doubling your pawns.
Grab any open files for your Rooks: When pawns are traded, they leave behind open files. Be quick and place a Rook behind an open file to gain control over the entire file. Later you could also double Rooks on the same file for a powerful attack.
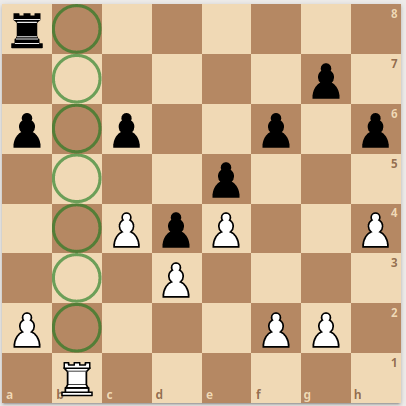
Avoid exchanging the fianchettoed Bishop: The fianchettoed Bishop is supposed to defend the weaker squares around the King and control a long diagonal. You shouldn’t exchange it in early or mid game if that’s an option.
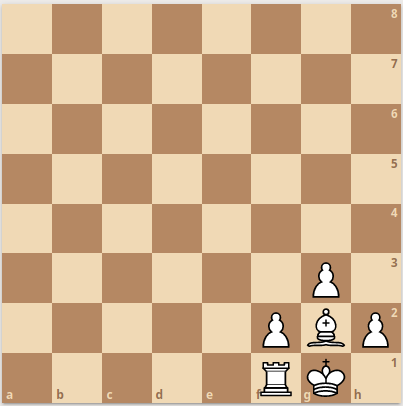
Know thy Bishops – Bad Bishop vs good Bishop: A bad Bishop is one that’s obstructed by your own pawns, ending up looking like a pawn itself (and not controlling much space). A good bishop covers a nice long diagonal.
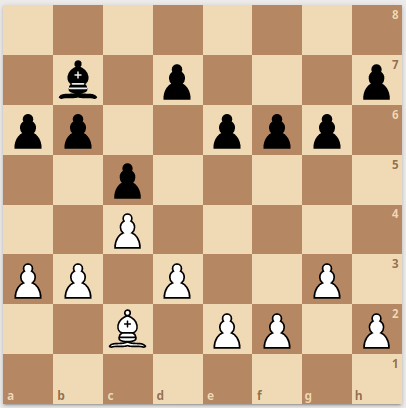
Bishops and Knights are equal, except…: Bishops and Knights are both 3 points, but Bishops are usually preferred when the board has few obstacles, while Knights are preferred when the position is very tight. In general, a Bishop pair is always something you’d want to save if you can.
Be mindful of forks: Forks come when two pieces are attacked by an opponent’s piece and we lose one of them anyhow. Knight forks are common and can be tricky to spot. A fork that comes with an attack on the King and the Queen is called a royal fork.
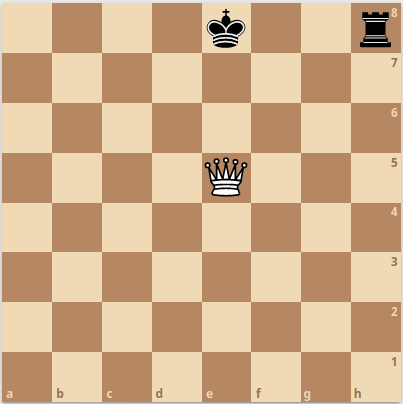
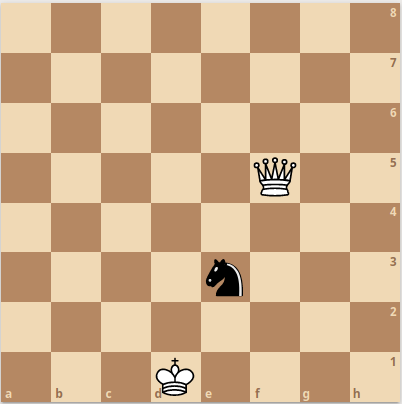
Next steps
From here, you should learn some of the openings and endgames. Openings will help you quicky get to a position where all of your pieces are mobilized and ready, and also know what’s on your opponent’s mind depending on what they play.
Endings are important to know how to actually win most games. Many games might not end with you having a bunch of heavy pieces storming your opponent’s king. On the other hand it would be quiet and tactical, and you should know how to best make use of the couple of minor pieces and pawns that are on the board.
In closing
Hope you enjoyed this article on some beginner tips for levelling up in Chess. If you’d like to play Chess with me, challenge me on lichess at lichess.org/@/abhn.
Thank you for reading!